Show Notes
Hosts: Jeff Cunningham and Ryan Harris
Guest: Brent Shaw, Senior Director of Scientific and Content Engineering at DTN
Description: Weather and climate is big business, but business value creation is not always created with only running the best weather models. Instead, value is generated when weather companies solve customer problems. Learn how Brent Shaw delivers relevant, reliable, and scalable solutions with an understanding a customer's "five whys". In this episode we discuss the latest trends in weather modeling, Brent's experience with the "bird poo algorithm", and many more commercial weather insights. Throughout, Brent gives sage advice for early weather career professionals seeking to work in the military, government, or commercial sectors. LISTEN HERE
References:
- Climate models warn of possible “super El Nino” this summer (Guardian)
- Climate change could be juicing baseball homeruns (NPR)
- India braces for season’s first heatwave (Weather Channel)
- ForecastWatch
- The same tech used by self-driving cars is now used to measure snow (Spectrum News)
- Historic rain in South Florida causes severe flooding, closing schools and Fort Lauderdale's airport (CNN)
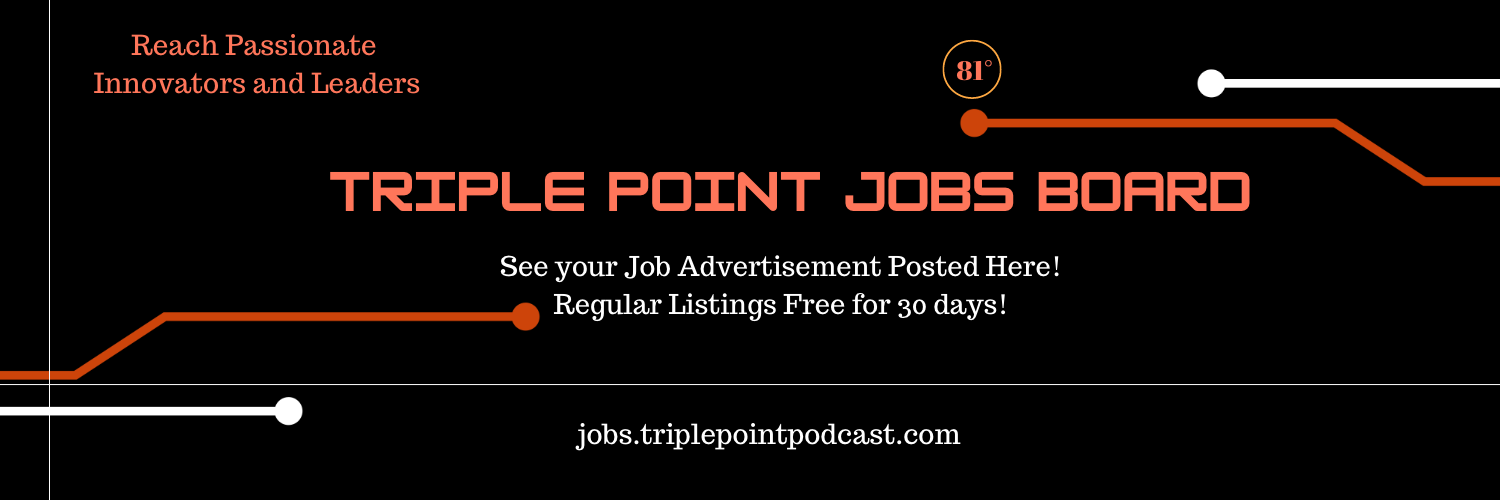
Journey to becoming a commercial weather innovator
(13:11)
How meteorologists can create commercial value
(20:31)
Being reliable, relevant, scalable is really where the money is
(27:16)
Trends in commercial weather modeling
(28:21)
A good place for machine learning is where we know there's a connection between the atmosphere, the ocean, and some impact the customer cares about
(33:52)
Solving problems through the socioeconomic lens
(36:46)
How non-weather/climate communities can start to observe their data better so that we can do machine learning and AI better
(39:14)
The role of government and commercial weather services
(43:21)
The bird poo algorithm!
(45:38)
Does a weather and climate company need to own the vertical?
(52:55)
The gamer changer "SpaceX" moment for weather commercialization
(59:44)
Thoughts for early career professionals
(1:01:45)
Member discussion: